CARG HUB: AI for bioreactors
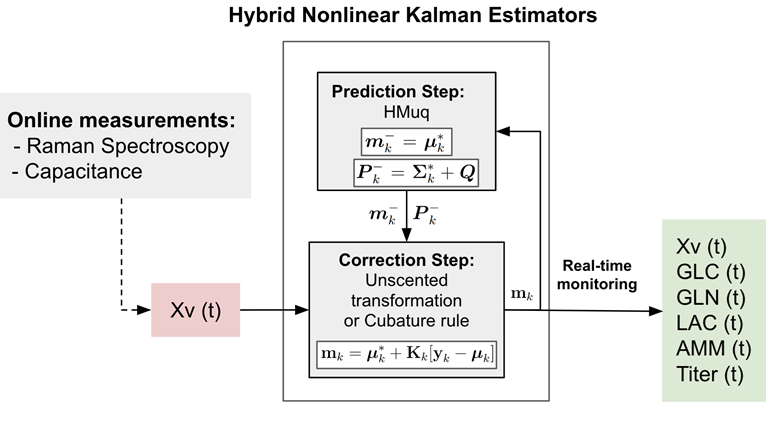
This research is all about improving how we monitor bioprocesses in the biopharmaceutical industry, making it faster and less expensive. The project focuses on developing advanced tools called nonlinear Kalman estimators (NKEs) to tackle these issues. Specifically, it aims to solve three big challenges: estimating important process parameters in complex biological systems, automatically tuning these estimation tools for different production conditions, and making the estimators more adaptable and accurate for different types of processes.
Research Papers
- C. Iglesias Jr, S. A. de Outeiro, C. Miceli de Farias and M. Bolic, Two Students: Enabling Uncertainty Quantification in Federated Learning Clients, NeurIPS 2024 Workshop on Bayesian Decision-making and Uncertainty, 2024.
- - Description: This paper is about novel Bayesian approaches for federated learning.
- Iglesias Jr, CF, Bolic, M. How Not to Make the Joint Extended Kalman Filter Fail with Unstructured Mechanistic Models. Sensors, 2024.
- - Description: Addresses the problems in joint estimation of states and parameters with an extended Kalman filter.
- Iglesias, CF, Ristovski, M, Bolic, M, Cuperlovic-Culf, M. rAAV Manufacturing: The Challenges of Soft Sensing during Upstream Processing. Bioengineering, 2023.
- - Description: This is a survey paper about machine learning models for rAAV manufacturing.
- Iglesias Jr, CF, Xu, X, Mehta, V, Akassou, M, Venereo-Sanchez, A, Belacel, N, Bolic, M. Monitoring the Recombinant Adeno-Associated Virus Production using Extended Kalman Filter. Processes, 2022.
- - Description: This paper monitors the rAAV production using the online viable cell density measurements and estimating the other state variables measured at a low frequency.
- Iglesias, J. C., Mehta, V., Venereo-Sanchez, A., Xu, X., Robitaille, J., Voyer, R., others. Handling Massive Proportion of Missing Labels in Multivariate Long-Term Time Series Forecasting. Journal of Physics: Conference Series, 2090 012170,2021.
- - Description: This paper handles situation where there are sparse labels for training machine learning forecast models for time series.