CARG AI4UAV: AI-based Counter UAS Systems
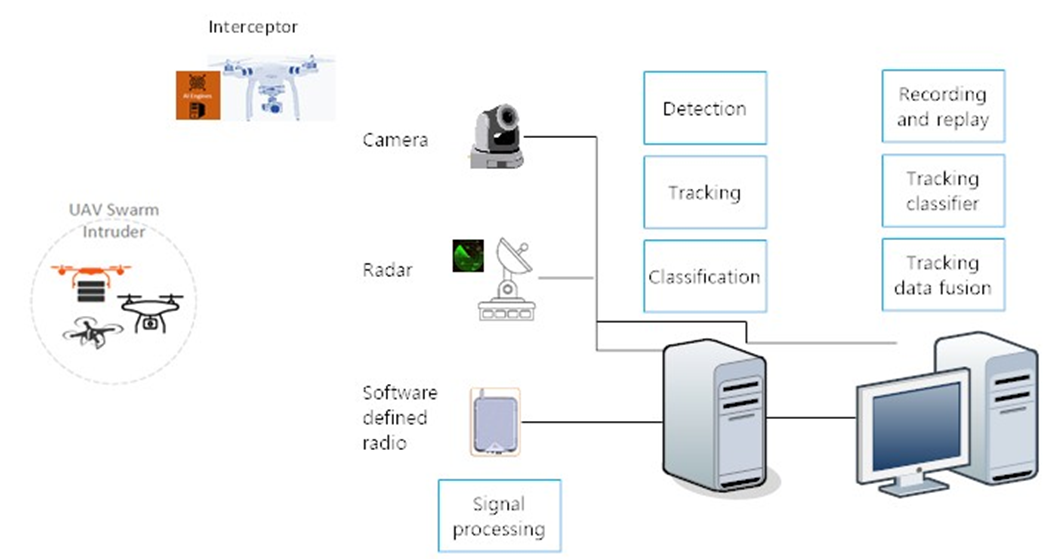
Rapid advancements in UAV technology demand sophisticated detection and response systems to address threats in national defense, security, and airspace safety. The CARG AI4UAV team utilizes their expertise in radar, statistical signal processing, machine learning, and computer vision to develop systems capable of detecting, classifying, and tracking UAVs using both ground and airborne sensors.
Key aspects of our research include the development of resilient AI systems that maintain reliability despite domain shifts and uncertainties. The system architecture integrates ground sensors like radar and PTZ cameras with interceptor UAVs to provide early warning, detection, tracking, and payload classification. The team tackles challenges such as long-distance object detection, ensuring model robustness with unseen data, and multi-sensor fusion. Our work combines modeling and simulations with extensive data collection. This work is supported by funding from NRC and NSERC.
Research Papers
- Mehta, V, Dadboud, F, Bolic, M, Mantegh, I. A Deep Learning Approach for Drone Detection and Classification Using Radar and Camera Sensor Fusion. 2023 IEEE Sensors Applications Symposium (SAS), 2023.
- - Description: A Deep Learning Approach for Drone Detection and Classification Using Radar and Camera Sensor Fusion published in 2023 IEEE Sensors Applications Symposium (SAS).
- F. Dadboud, H. Azad, V. Mehta, M. Bolic, I. Mantegh, DrIFT: Autonomous Drone Dataset with Integrated Real and Synthetic Data, Flexible Views, and Transformed Domains,IEEE/CVF Winter Conference on Applications of Computer Vision (WACV) 2025.
- - Description: This paper is about studying drone detection under different types of domain shift.
- N. Bowness, UAV Object Tracking with Modular Architecture, University of Ottawa, 2024.
- - Description: Signal processing and machine learning for fusion of airborne camera and radar data.
- Kaza, K, Mehta, V, Azad, H, Bolic, M, Mantegh, I. An Intent Modeling and Inference Framework for Autonomous and Remotely Piloted Aerial Systems. arXiv preprint arXiv:2409.08472, 2024.
- - Description: An Intent Modeling and Inference Framework for Autonomous and Remotely Piloted Aerial Systems published in arXiv preprint arXiv:2409.08472.
- H. Azad, V. Mehta, F. Dadboud, M. Bolic and I. Mantegh, Air-to-Air Simulated Drone Dataset for AI-powered problems, 2023 IEEE/AIAA 42nd Digital Avionics Systems Conference (DASC), Barcelona, Spain, 2023, pp. 1-7, doi: 10.1109/DASC58513.2023.10311339.
- - Description: This paper presents a comprehensive multi-view air-to-air simulated vision drone dataset.
- Patel, V, Mehta, V, Bolic, M, Mantegh, I. A Hybrid Framework for Object Distance Estimation using a Monocular Camera. 2023 IEEE/AIAA 42nd Digital Avionics Systems Conference (DASC), 2023.
- - Description: A deep learning method for estimating the distance of intruder UAVs using a single camera.
- Mehta, V., Bolic, M., Mantegh, I., Vidal, C.. Tracking and Classification of Drones and Birds at a Far Distance Using Radar Data, 2021.
- - Description: Distinguishing between birds and drones based on their motion patterns.
- X. Zhang, V. Mehta, M. Bolic and I. Mantegh, Hybrid AI-enabled Method for UAS and Bird Detection and Classification, IEEE International Conference on Systems, Man, and Cybernetics (SMC), 2020.
- - Description: A novel approach combining IMM filters and LSTM-based RNNs achieved 99.3% accuracy in classifying drones versus birds using synthetic trajectory data.